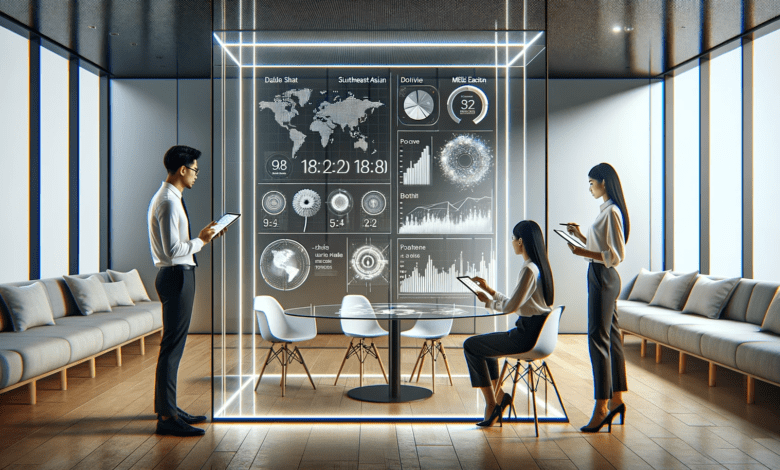
Data has become an increasingly valuable asset for businesses, driving innovation, revenue generation, and competitive advantage. To fully harness the power of data, organizations must understand its true value and prioritize their efforts accordingly. In this comprehensive guide, I will explore various methodologies for measuring the value of data and how they can be applied to drive business success. From understanding the foundational elements of data valuation to exploring different valuation methods, I will provide insights and practical strategies to help organizations unlock the potential of their data assets.
An Introduction to Data Valuation
In today’s data-driven world, managing data effectively is crucial for businesses. However, to truly manage data, organizations must first understand its value. Data valuation is the process of determining the worth of data assets and prioritizing them based on their value. As businesses increasingly turn to data monetization, it becomes essential to identify the most valuable data assets to optimize return on investment.
Data valuation is a critical step towards becoming a data-driven business. Understanding the value of data offers several advantages:
- Informed Decision-Making: Valuable data provides valuable insights that inform decision-making processes, leading to better outcomes.
- Resource Allocation: Knowing the value of data allows organizations to allocate limited resources to areas that generate the most value, maximizing return on investment.
- Monetary Value: Assigning a monetary value to data assets helps organizations compare them with other assets in the business, facilitating better financial decision-making.
- Data Culture: Communicating the monetary value of data assets helps foster a data-centric culture within the organization, where data is recognized as a valuable asset.
- Boosting Morale: Understanding the value of data boosts the morale of the data team, positioning them as custodians of valuable assets and experts in their field.
Data valuation involves evaluating the various factors that contribute to the value of data assets. These factors include data quality, relevance, uniqueness, volume, diversity, reliability, and security. Each of these factors plays a crucial role in determining the overall value of the data asset.
- Data Quality: High-quality data that is accurate, complete, and up to date is more valuable than low-quality data. Data users rely on the quality of the data to make informed decisions and derive meaningful insights. Therefore, data sets that score high on data quality are likely to have a higher valuation.
- Data Relevance: The relevance of data refers to its applicability to a wide range of users and its broad usefulness. Data that has broad applicability and can be used in various contexts is more valuable than data that is relevant only to a niche market. Organizations should assess the relevance of their data assets to determine their value.
- Data Uniqueness: The uniqueness of data refers to its exclusivity or difficulty in obtaining it. Data that is exclusive or difficult to obtain is more valuable than data that is widely available or easily accessible. Unique data sets provide organizations with a competitive advantage and can command a higher valuation.
- Data Volume: The volume of data refers to the amount of information contained within a data set. Large datasets that contain a significant amount of information are more valuable than smaller datasets. The volume of data contributes to its potential for generating insights and supporting decision-making processes.
- Data Diversity: Data diversity refers to the variety and range of information contained within a data set. Datasets that contain a diverse range of information, such as data from different sources or in different formats, are more valuable than datasets that are limited in scope. Data diversity enhances the potential for generating comprehensive insights and addressing complex business challenges.
- Data Reliability: Reliable data refers to data that is obtained from trustworthy sources and has been verified for accuracy and authenticity. Data that is reliable is more valuable than data that is unverified or unreliable. Organizations rely on reliable data to make critical business decisions and mitigate risks.
- Data Security: Data security refers to the measures in place to protect data assets from unauthorized access, breaches, or other threats. Data that is well-protected and secure is more valuable than data that is vulnerable to security risks. Organizations must prioritize data security to safeguard their data assets and maintain their value.
How to Calculate the Value of Data
Calculating the value of data requires a systematic approach. While there are various methodologies available, understanding your data assets and how they drive value is the first step. Let’s explore some of the major data valuation methodologies:
Method 1: Cost Value Method
The cost-based approach involves determining the cost of acquiring, processing, storing, and managing data to determine its value. This approach considers the expenses associated with data collection, storage infrastructure, data management tools, and data governance processes. By calculating the costs incurred to acquire and maintain the data, organizations can assess its value. Several data valuation techniques, such as Daniel Moody’s 7 Laws of Information and Glue Reply Valuation Technique, use this method. While it provides a partial understanding of data value by capturing costs, it falls short in capturing the broader economic picture and how data translates into business value.
Method 2: Market Value Approach
The market value approach assesses the value of data by examining what others pay for comparable data on the open market. However, this methodology has limitations. Some data may not be traded, and there may be no comparable examples available. Additionally, price does not necessarily equate to value, and efficient markets for data are still developing.
Method 3: Economic Value Approach
The economic value approach focuses on the impact of data on the bottom line of the business. It includes methods such as income or utility valuation, which tracks the value added by data to specific business functions or use cases. A derivative of this method is the so-called “income-based approach”. The income-based approach involves estimating the future revenue that can be generated by using a particular dataset to determine its value. This approach focuses on the potential value added by the data in generating business outcomes and revenue streams. By projecting the impact of the data on the organization’s bottom line, organizations can assess its value. However, this approach may be challenging as it requires accurate revenue projections and assumptions about the data’s contribution to business outcomes.
Method 4: Stakeholder Value Approach
The stakeholder value approach considers the value created for all stakeholders, including shareholders, customers, employees, suppliers, communities, and the environment. It aligns with the shift towards stakeholder capitalism and environmental, societal, and governance (ESG) factors. This approach provides a more holistic understanding of data value, considering the broader context of value creation.
One way to derive the stakeholder value of data is by employing the so-called “survey valuation approach”, which involves conducting surveys or other direct research methods to determine how much people would be willing to pay for a particular dataset. This method relies on gathering feedback and opinions from potential data users or customers to estimate the value of the data. By understanding the perceived value of the data, organizations can assess its market demand and potential value. However, this approach may be subjective and dependent on the survey participants’ preferences and biases.
Method 5: Replacement Pricing Approach
The replacement pricing approach involves analyzing the prices of goods or services that are influenced by a particular dataset to determine its value. This approach considers the value added by the data in enabling or enhancing certain products or services. By examining the prices of goods or services that rely on the data, organizations can estimate its value. However, this approach may be limited to specific industries or applications.
Effective Implementation of a Data Valuation Strategy
Implementing a data valuation strategy requires several key capabilities or building blocks. These building blocks provide the foundation for successful data management and decision-making. Let’s explore the five essential building blocks:
1. Data Culture: Cultivating a data culture within the organization is crucial. This involves fostering a mindset that values and encourages the use of data for improved performance.
2. Data Literacy: Enhancing data literacy across the organization is essential for effective data management. This involves providing formal training programs and hands-on experience to improve understanding and communication of data and insights.
3. Quality Data: Ensuring data quality is a fundamental aspect of data valuation. Organizations must focus on data profiling, minimalism, and robust data integration capabilities to improve data quality and suitability for decision-making.
4. Tools and Technology: Utilizing appropriate data analytics tools and technology is necessary for efficient data management. These tools help streamline processes, maintain data flow, and enhance scalability, speed, and security.
5. Data Governance: Implementing data governance practices is critical to securely manage data quality throughout its lifecycle. This ensures that the right people are securely managing the right data in the right manner.
Challenges in Data Valuation
Data valuation can be a complex and challenging exercise for organizations. There are several practical challenges that organizations may face when valuing their data assets. These challenges include the lack of standardized valuation methods, a lack of understanding of the data, difficulty in quantifying the value of intangible data assets, rapidly changing market dynamics, lack of transparency and standardization in data markets, and data privacy and security concerns.
- Lack of Standardized Valuation Methods: One of the primary challenges in data valuation is the lack of standardized approaches and methodologies. There is no one-size-fits-all method for valuing data, and different valuation methods may yield different results. This lack of standardization makes it difficult for organizations to compare the value of different data assets or establish a clear valuation framework.
- Lack of Understanding of the Data: Valuing data assets requires a deep understanding of the data itself, including its characteristics, quality, relevance, and potential uses. Organizations may struggle to accurately value their data assets if they lack this understanding. It is crucial for organizations to invest in data governance and data management practices to gain insights into their data assets.
- Difficulty in Quantifying the Value of Intangible Data Assets: Data assets may have intangible value, such as the value of insights that can be generated from the data. These intangible values can be challenging to quantify and may require more subjective valuation methods. Organizations need to consider the potential intangible value of their data assets when performing valuations.
- Rapidly Changing Market Dynamics: The value of data assets can change rapidly due to changes in market dynamics, such as shifts in consumer behavior or changes in technology. This dynamic nature of the data market makes it challenging to perform accurate and timely valuations. Organizations need to stay updated on market trends and adapt their valuation approaches accordingly.
- Lack of Transparency and Standardization in Data Markets: Data markets are often fragmented and lack transparency, making it difficult for organizations to access and value data assets. The lack of standardized pricing and trading mechanisms for data assets further complicates the valuation process. Organizations need to navigate these challenges by building partnerships and networks to access relevant data assets and market information.
- Data Privacy and Security Concerns: Valuing data assets requires access to sensitive data, which may pose privacy and security risks if not handled appropriately. Organizations must ensure that proper data protection measures are in place, including data anonymization, encryption, and access controls. Addressing data privacy and security concerns is crucial for maintaining the value and integrity of data assets.
Conclusion
Effective communication is vital to maintain commitment and drive successful data initiatives. Translating data value into business value involves aligning metrics and measurements with stakeholders’ interests. By linking progress metrics with impact metrics and aligning them with broader business goals, organizations can demonstrate the tangible value of data and drive better management and decision-making.
Data valuation is a crucial step in unlocking the potential of data assets for business success. By understanding the value of data and implementing effective data valuation methodologies, organizations can optimize their data-driven strategies, allocate resources wisely, and create a data-centric culture. The building blocks of data culture, data literacy, quality data, tools and technology, and data governance provide the foundation for successful implementation. Through effective communication and translation of data value into business value, organizations can harness the power of data to drive innovation, revenue generation, and competitive advantage in today’s data-driven business landscape.
If you’re interested in unlocking the benefits of data valuation for your organization, feel free to contact us. We can help you navigate the complexities of data valuation and develop strategies to maximize the value of your data assets.
Note: The information provided in this article is for educational purposes only and should not be considered as financial or legal advice.